2022
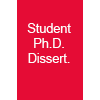
J. Steiner
Locomotion and Steering of a Magnetic-electroactive Endoluminal Soft Robot PhD Thesis
2022.
@phdthesis{SteinerJ_2022_PHD,
title = {Locomotion and Steering of a Magnetic-electroactive Endoluminal Soft Robot},
author = {J. Steiner},
year = {2022},
date = {2022-08-12},
keywords = {},
pubstate = {published},
tppubtype = {phdthesis}
}
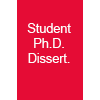
W. S. Nagel
Long-Range Low-Coupling Dual-Stage Nanopositioning: Design and Control for High-Speed Atomic Force Microscopy PhD Thesis
2022.
@phdthesis{NagelW_2022_PHD,
title = {Long-Range Low-Coupling Dual-Stage Nanopositioning: Design and Control for High-Speed Atomic Force Microscopy},
author = {W. S. Nagel},
year = {2022},
date = {2022-04-18},
keywords = {},
pubstate = {published},
tppubtype = {phdthesis}
}
2020
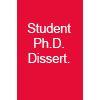
Joseph R. Bourne
Decentralized multi-agent information theoretic target localization and estimation: finding and predicting chemical gas leaks PhD Thesis
University of Utah, 2020.
@phdthesis{BourneJR_2020b,
title = {Decentralized multi-agent information theoretic target localization and estimation: finding and predicting chemical gas leaks},
author = {Joseph R. Bourne},
year = {2020},
date = {2020-06-13},
urldate = {2020-06-13},
school = {University of Utah},
abstract = {The goal of this dissertation is to quickly and autonomously localize and estimate an unknown target, such as a chemical leak, using a team of mobile robots. Accidental or malicious release of chemical, biological, radiological, nuclear, or explosive (CBRNE) substances can have devastating effects on humans, animals, infrastructure, and the environment. Thus, fast and accurate localization and estimation of a contaminant release are crucial to saving lives and minimizing damage. To achieve the goal, stochastic estimation and motion planning algorithms are developed, where the performance of two distinct methods for coordinating the mobile robot team are studied. First, a new non-parametric Bayesian-based motion planning algorithm for autonomous plume source term estimation (STE) and source seeking (SS) is developed. Robots coordinate their movements by parsing the belief into multiple modes and they investigate these modes through model-based bio-inspired SS actions. Simulation and experimental results show consistently that the coordinated Bayesian-based STE and SS algorithm outperforms traditional bio-inspired SS and raster-scanning methods, where performance is approximately twice as fast as the uncoordinated case. Second, a new decentralized multi-agent information-theoretic (De-
MAIT) control algorithm that leverages Bayesian estimation and guides robots to minimize uncertainty is developed. The algorithm consists of: (1) a non-parametric Bayesian estimator,
(2) an information-theoretic trajectory planner that generates “informative trajectories” for an agent to follow, and (3) a controller and collision avoidance algorithm that ensure agents follow the trajectory as closely as possible in a safe manner. Simulation results show that the DeMAIT algorithm’s average localization success rate is higher and more robust to changes in the source location, robot team size, and search area size, compared to the coordinated bio-inspired method. Outdoor field experiments are conducted using a team of custom-built aerial robots equipped with gas concentration sensors to estimate and find the source of a propane gas leak to demonstrate efficacy of the DeMAIT algorithm.},
keywords = {},
pubstate = {published},
tppubtype = {phdthesis}
}
MAIT) control algorithm that leverages Bayesian estimation and guides robots to minimize uncertainty is developed. The algorithm consists of: (1) a non-parametric Bayesian estimator,
(2) an information-theoretic trajectory planner that generates “informative trajectories” for an agent to follow, and (3) a controller and collision avoidance algorithm that ensure agents follow the trajectory as closely as possible in a safe manner. Simulation results show that the DeMAIT algorithm’s average localization success rate is higher and more robust to changes in the source location, robot team size, and search area size, compared to the coordinated bio-inspired method. Outdoor field experiments are conducted using a team of custom-built aerial robots equipped with gas concentration sensors to estimate and find the source of a propane gas leak to demonstrate efficacy of the DeMAIT algorithm.
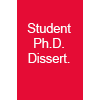
Xiang He
Modeling and Control of In-Ground-Effect on Rotorcraft Unmanned Aerial Vehicles PhD Thesis
University of Utah, 2020.
@phdthesis{HeX_2020_PhD,
title = {Modeling and Control of In-Ground-Effect on Rotorcraft Unmanned Aerial Vehicles},
author = {Xiang He},
year = {2020},
date = {2020-01-11},
school = {University of Utah},
abstract = {The goal of this dissertation is to model and control the behavior of in-ground-effect (IGE) on multirotor unmanned aerial vehicles (UAVs). Ground effect, or rotor IGE, is a common phenomenon experienced by rotorcraft aerial vehicles when taking off, landing on, hovering around, or flying near surfaces or obstacles. Rotor IGE is caused by aerodynamic interaction between the rotor wake and the nearby obstacle. In particular, the deformed wake causes changes in the induced velocity, which leads to drastic changes in rotor thrust and torque that make flight control in confined spaces difficult and challenging. Many of the existing models for IGE are based on work on helicopters from the early 1940s and more so from the 1950s. Many of these models, unfortunately, are not directly applicable to smaller rotorcraft aerial vehicles due to the assumptions that were made. Also, many of these models suffer from singularities at certain heights, and many of them are computationally heavy. The contributions of this dissertation are computationally-light and accurate models of IGE and the development of feedback controllers that are effective at handling IGE. First, a quasi-steady IGE model for a single rotor that predicts a finite maximum IGE thrust ratio is developed. An empirical approach is used to establish the base exponential function in the quasi-steady model, followed by exploiting blade element theory (BET) and the semipositive induced velocity assumption to relate two IGE model coefficients to blade geometry. The changes in the rotor IGE for various multirotor configurations are studied, characterized, and modeled with respect to the number of rotors, rotor rotation direction, and minimum rotor tip-to-tip distance. The quasi-steady model also incorporates a newly-discovered fountain-vortex thrust loss effect. The quasi-steady model is experimentally validated for off-the-shelf and variable pitch propellers. Second, the quasi-steady model is extended to capture dynamic IGE by considering vehicle flight states and the partial ground effect where a portion of a rotor operates within the ground-effect region. More specifically, using blade element theory, rotor IGE thrust ratios in forward and axial flight are derived as a function of the advance ratio and climbing speed in the IGE regime. A rotation-based IGE test stand that simulates forward flight is created and used to characterize dynamic IGE and to validate the analytical results. The advance ratio, rotor disk angle of attack (AOA), and various multirotor configurations (transverse and tandem rotor) are investigated using the test stand. The partial ground effect is empirically characterized, and the behavior is incorporated into the dynamic IGE model. Finally, the developed IGE models are exploited for vehicle motion control to account for IGE on a quadrotor helicopter (quadcopter) aerial vehicle flying near the ground surface. Specifically, a feedback-based nonlinear disturbance observer controller and a feedforward IGE compensator are designed, simulated, and implemented. Simulation and experimental results validate the effectiveness of the IGE model and flight controller to compensate for IGE when flying close to the ground.},
keywords = {},
pubstate = {published},
tppubtype = {phdthesis}
}
2019
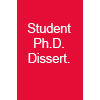
Dejun Guo
Dynamic and Aggressive Image-Based Flying in GPS-Denied Environments: Estimation, Motion Planning, and Control PhD Thesis
University of Utah, 2019.
@phdthesis{GuoD_2019_PhD,
title = {Dynamic and Aggressive Image-Based Flying in GPS-Denied Environments: Estimation, Motion Planning, and Control},
author = {Dejun Guo},
year = {2019},
date = {2019-05-18},
school = {University of Utah},
abstract = {The goal of this dissertation is to enable an aerial robotic system (including an aircraft with a cable-suspended payload) to fly autonomously in GPS-denied environments through vision from a single monocular camera. To achieve this goal, new estimation, motion planning, and control algorithms that exploit the image-based visual-servo framework are developed. Rigorous stability analysis based on the Lyapunov approach is also presented for the developed control systems. The image-based framework is of interest because of its robustness to image noise and lower computational demand compared to position-based techniques where pose estimation is required. However, this research tackles inherent challenges including nonlinear dynamics, singularity issues, and complex stability analysis for cases with relaxed constraints on initial estimation errors, vehicle position, and height. The resulting theoretical outcomes are validated experimentally by showing demonstrations of aerial-robot assisted operations related to emergency response, search and rescue, and package delivery in GPS-denied environments, such inside of buildings or in urban canyons where global vehicle localization and control schemes are ineffective or impractical. Firstly, a new kinematic image-based control algorithm using a mobile overhead camera for aerial robots is developed. The control algorithm exploits adaptation to compensate for uncertainties in the camera parameters and depth information, and repetitive control is used to reject inherent periodic tracking errors in the image plane. Stability analysis in the Lyapunov sense is shown. Both simulations and physical experiments are provided for a quadcopter to demonstrate the approach. Secondly, a new nonlinear flight control scheme that combines a vision-based closed-loop observer with a backstepping-based controller using an onboard camera and the inertial measurement unit (IMU) is developed. This new approach is computationally efficient and asymptotically stable. Flight tests are conducted to validate the algorithm’s capabilities for take-off, to hover, to track trajectory, and landing. Finally, a new flight control scheme that combines an image-based position controller and a quaternion-based attitude controller are created that enables and aerial robot to fly aggressively through several narrow windows without knowledge of the robot's position and window size, and the approach is extended to control the motion of a cable-suspended payload for package delivery. Both simulations and physical experiments demonstrate that the approaches are capable of flying into and out of a small house and picking up and transporting several packages with unknown mass.},
keywords = {},
pubstate = {published},
tppubtype = {phdthesis}
}
2018
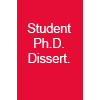
James D. Carrico
3D printing, performance characterization, and machine learning control of ionic polymer-metal composite actuators with applications in soft robotics PhD Thesis
University of Utah, 2018.
@phdthesis{CarricoJD_2018_PHD,
title = {3D printing, performance characterization, and machine learning control of ionic polymer-metal composite actuators with applications in soft robotics},
author = {James D. Carrico},
year = {2018},
date = {2018-09-17},
school = {University of Utah},
keywords = {},
pubstate = {published},
tppubtype = {phdthesis}
}
2016
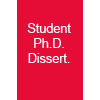
D. Bareiss
University of Utah, 2016.
@phdthesis{BareissD_2016b,
title = {Model-based collision avoidance for dynamic single- and multi-robot systems: theory and application in ground and aerial robots},
author = {D. Bareiss},
url = {http://www.kam.k.leang.com/academics/pubs/2016BareissDissertation.pdf},
year = {2016},
date = {2016-06-07},
school = {University of Utah},
abstract = {This dissertation solves the collision avoidance problem for single and multi-robot systems where dynamic effects are significant. In many robotic systems (e.g., highly maneuverable and agile unmanned aerial vehicles) the dynamics cannot be ignored and collision avoidance schemes based on kinematic models can result in collisions or provide limited performance, especially at high operating speeds. Herein, real-time, model-based collision avoidance algorithms that explicitly consider the robots' dynamics and perform real-time input changes to alter the trajectory and steer the robot away from potential collisions are developed, implemented, and verified in simulations and physical experiments. Such algorithms are critical in applications where a high degree of autonomy and performance are needed, for example in robot-assisted first response where aerial and/or mobile ground robots are required to maneuver quickly through cluttered and dangerous environments in search of survivors. Firstly, the research extends reciprocal collision avoidance to robots with dynamics by unifying previous approaches to reciprocal collision avoidance under a single, generalized representation using control obstacles. In fact, it is shown how velocity obstacles, acceleration velocity obstacles, continuous control obstacles, and linear quadratic regulator (LQR)-obstacles are special instances of the generalized framework. Furthermore, an extension of control obstacles to general reciprocal collision avoidance for non-linear,
non-homogeneous systems where the robots may have different state spaces and different non-linear equations of motion from one another is described. Both simulations and physical experiments are provided for a combination of differential-drive, differential-drive with a trailer, and car-like robots to demonstrate that the approach is capable of letting a non-homogeneous group of robots with non-linear equations of motion safely avoid collisions at real-time computation rates. Secondly, the research develops a stochastic collision avoidance algorithm for a tele-operated unmanned aerial vehicle (UAV) that considers uncertainty in the robot's dynamics model and the obstacles' position as measured from sensors. The model-based automatic collision avoidance algorithm is implemented on a custom-designed quadcopter UAV system with on-board computation and the sensor data is processed using a split-and-merge segmentation algorithm and an approximate Minkowski difference. Flight tests are conducted to validate the algorithm's capabilities for providing tele-operated collision-free operation. Finally, a set of human subject studies are performed to quantitatively compare the performance between the model-based algorithm, the basic risk field algorithm (a variant on potential field), and full manual control. The results show that the model-based algorithm performs significantly better than manual control in both the number of collisions and the UAVs average speed, both of which are extremely vital, for example, for UAV-assisted search and rescue applications. Compared to the potential-field based algorithm, the model-based algorithm allowed the pilot to operate the UAV with higher average speeds.},
keywords = {},
pubstate = {published},
tppubtype = {phdthesis}
}
non-homogeneous systems where the robots may have different state spaces and different non-linear equations of motion from one another is described. Both simulations and physical experiments are provided for a combination of differential-drive, differential-drive with a trailer, and car-like robots to demonstrate that the approach is capable of letting a non-homogeneous group of robots with non-linear equations of motion safely avoid collisions at real-time computation rates. Secondly, the research develops a stochastic collision avoidance algorithm for a tele-operated unmanned aerial vehicle (UAV) that considers uncertainty in the robot's dynamics model and the obstacles' position as measured from sensors. The model-based automatic collision avoidance algorithm is implemented on a custom-designed quadcopter UAV system with on-board computation and the sensor data is processed using a split-and-merge segmentation algorithm and an approximate Minkowski difference. Flight tests are conducted to validate the algorithm's capabilities for providing tele-operated collision-free operation. Finally, a set of human subject studies are performed to quantitatively compare the performance between the model-based algorithm, the basic risk field algorithm (a variant on potential field), and full manual control. The results show that the model-based algorithm performs significantly better than manual control in both the number of collisions and the UAVs average speed, both of which are extremely vital, for example, for UAV-assisted search and rescue applications. Compared to the potential-field based algorithm, the model-based algorithm allowed the pilot to operate the UAV with higher average speeds.
2011
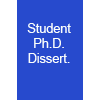
Yingfeng Shan
Repetitive control for hysteretic systems: theory and application in piezo-based nanopositioners PhD Thesis
Univesity of Nevada, Reno, 2011.
@phdthesis{ShanY_2011b,
title = {Repetitive control for hysteretic systems: theory and application in piezo-based nanopositioners},
author = {Yingfeng Shan},
url = {http://www.kam.k.leang.com/academics/pubs/ShanY_2011_Dissertation.pdf},
year = {2011},
date = {2011-12-17},
address = {Reno, Nevada 89557-0312},
school = {Univesity of Nevada, Reno},
keywords = {},
pubstate = {published},
tppubtype = {phdthesis}
}
2004
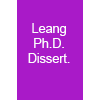
K. K. Leang
University of Washington, 2004.
@phdthesis{LeangKK_2004b,
title = {Iterative learning control of hysteresis in piezo-based nanopositioners: theory and application in atomic force microscopes},
author = {K. K. Leang},
url = {http://www.kam.k.leang.com/academics/pubs/KamKLeangPhDDec2004.pdf},
year = {2004},
date = {2004-01-01},
address = {Seattle, WA},
school = {University of Washington},
keywords = {},
pubstate = {published},
tppubtype = {phdthesis}
}